SoS #7: The Sustainability Data Collection Problem
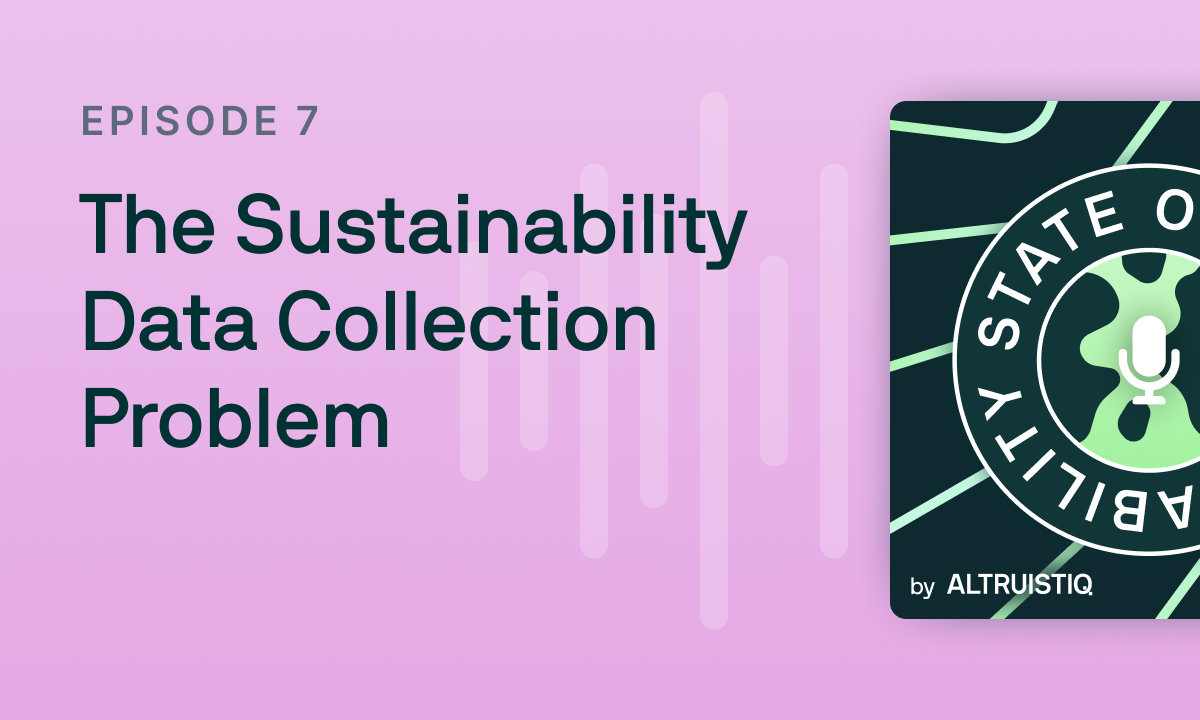
What you'll learn
Prefer to watch?
Transcript:
Isobel: Hi, everyone. Welcome to State of Sustainability, where we unwrap and unpack the stickiest of sustainability issues.
Today, we're going to talk about primary and secondary data off the back of quite a lively LinkedIn debate. So for all of those who were included, this, this is for you. We might name names further on in the discussion, but really exciting today. We're joined by Toby, VP of data at Altruistiq, and of course, Saif, and I think we're going to just run down what even is primary data versus secondary data, what is the problem, and how do we actually put this into practice and implement it on a day-to-day.
So to start off, what is the problem? Saif, do you want to take the floor?
Saif: Well actually, Izzy, just to start off with sticky problems, I should warn you in our audience. And by the way, we normally don't do product placements, but I'll do one now, which is Toby and I have both just consumed a bun from home, basically.
So if anyone in London is familiar with buns from home, they are our favourite cinnamon buns. So we might be on a somewhat of a sugar high right now so if this sounds a little manic, it's not us, it's the sugar talking.
Isobel: Let's hope we don't have the sugar crash during this episode. Definitely. We need to make it through to the end at least.
Saif: Super excited, obviously, to kick off this topic. All right. I am intrigued, surprised, and somewhat excited by how much debate this topic raises, so I started talking about primary data versus secondary data probably three or four weeks ago. And the background for this is I was sort of noticing in my conversations with sustainability practitioners and also with let's say peripheral stakeholders.
The problem:
So I'm including corporate sustainability people, but also consultants, advisors, software stakeholders, etc. I was sort of noticing that there are these two schools of thought.
- Secondary Approach: No-touch solutions, you need complete, holistic, secondary data cover, and actually satellite data, some kind of advanced modelling, data science, AI, is going to allow you to solve everyone's Scope 3 problem by just having some sort of universal coverage of synthetic data of some sort.I think there's a set of vendors pitching that. And there's a set of stakeholders pitching that.
- Primary approach: You need universal primary data coverage. You need everyone entering data in somewhere, every supplier, every company entering in data for everything, every item that they purchase, every item that they produce.And that database should be made available to every company and every company can just pull data from there. And that solves everyone's scope three problem.
There's a different set of vendors behind that and a different set of stakeholders pitching that and the sustainability people working in in corporate teams basically get both sets of pitches and have to pick one and I started asking audiences at events where I would go to which of these two camps do you sort of fall into and I'm finding increasingly people falling into the primary data camp. I feel quite strongly that actually both camps are wrong.
What I've started saying at events that I go to is that you actually need hybrids. The reason for that is that if you look at, let's say, a few different factors, you look at cost, need, scalability and the sort of pragmatism of getting a solution out there.I don't think it's feasible for us to go out and get primary data coverage. It'll just never be cost-effective. And we'll talk about that a little more in this episode.
At the same time, I don't think secondary data can ever be as accurate as you would need it to be without some input of primary data.
And so my approach is always, we need a hybrid and we'll talk a bit more about that as well, but that's just to set the scene a bit, Izzy.
Isobel: Toby, from your perspective, would you agree that a hybrid approach is the way to go?
Toby: Yeah, absolutely, because I think trying to go out and getting primary data at scale is a huge undertaking, as Saif has mentioned, and it's going to be very hard to achieve.
And so you need to think about well, what do we actually need this data for? Which I know we're going to cover shortly later on in this session. You're not going to need for a lot of initiatives that you as a business are going to do, you're not going to need primary data immediately to be able to drive action, which is the most important point around collecting this data.What is good enough to start getting action out of it?
Saif: The majority of our audience is probably somewhat inclined to the primary data side and I put up a post on LinkedIn. I got a bunch of comments. I also got a comment from Martin Stuckey, co-founder of Systemiq, now co-founder of the land banking group and, and was one of my mentors at McKinsey and offering to wrestle on this topic and kind of championing the primary data side. So this is, this is also my, my response to that.
What is primary data capture:
I also think it's worth picking into what we think of as primary data capture. And right now, I think that most corporate sustainability professionals would assume that primary data is a company filling out a form.
I think that there's a hypothesis that that could work across the value chain. I think that is generally flawed for the global North. But I think it's impossible for the global South. And so the original discussion was around how if you take, let's say, farm-level data, agricultural data being some of the hardest here, and you imagine, let's say, a small farm, in the UK or in Europe, a small farm is still a corporate entity of some sort. It's usually a registered business. Everyone there has a certain level of tech literacy and ability to engage with some form of data provision and data capture. Whereas if you go to the global South, countries like Pakistan, where I'm from, you will never manage to capture this data in any way.
You're going to really struggle to get any form of engagement from farms for the simple reason that most of them are not literate as we would hold the bar, let's say here in the UK.
I actually started a farming business as my previous venture. We employed over 200 people on the farm. And it was a reasonably large farm.It was probably the largest flower farm in Pakistan. And we still really struggled with digitisation of any type. It wasn't that we were trying to solve for sustainability. We were trying to solve for like picking efficiency, picking the flowers. And we wanted to be able to get the pickers picking to give us some sense of how many flowers they picked.
And that was impossible because the pickers couldn't read or write. And there was no real way of getting that communication across. So then we thought, okay, the foreman managing the pickers should be able to tabulate in the basket, how many flowers came from each picker, per hour to get the picking efficiency. We couldn't get that to work either.
The hurdle on managing to get any kind of engagement from someone without a huge amount of effort. And by effort, I mean, literally training people on how to use digital tools, teaching them to kind of read and write and be numerate in some cases. This is not the job of food and apparel companies and brands to solve the education problem in emerging markets.
It can't be, it shouldn't be, it doesn't really make sense. It is a problem to be solved, but not by these businesses. Take the dairy example, which I was talking about on LinkedIn as another, another issue. Nestle is doing fantastic work on engaging dairy farms around the world, and kudos to them for that.
At the same time, I'm sure Nestle will be the first to agree that if you look at, let's say, dairy farmers in Pakistan and India, which are, by the way, the first and the third largest dairy producers in the world, I believe, by volume. About half, 50 percent of the output across both of those locations is smallholder dairy farmers.
Let me describe what we mean by smallholder dairy farmers. We're talking about a person who owns a cow. We're talking about a person who owns one animal, maybe two. If you think about the socioeconomic position of the kind of person who depends for their livelihood and their family's livelihood on one to two animals, with a yield, by the way, that is 30 percent or less of the yield you'd get in the Netherlands or Australia or the USA. That person is not going to be able to engage with any data capture form. So that format of primary data capture, where you ask the person to enter something, that is just not going to work for a large share of most materials that you buy from the Global South. And I use that as an extreme example just to illustrate the point.
I think the same difficulties are going to be there for the Global North as well, to a slightly lesser degree, and slightly less for some materials, slightly more for others, depends a little on location and size. But I just want to illustrate the problem.
Toby: I agree in that that kind of variety in data, in data literacy and digital literacy, if that's such a thing, really highlights the kind of complexity of the scale and the feasibility to roll out, you know, good primary data capture systems across, you know, across the supply chain. It's just not going to happen. And that's why you need to think about kind of coverage and materiality. Where is it important to get primary data where versus where is it good enough to get secondary data such that you can get the right level of actionability, but you don't necessarily need that immediately from that primary data.
Isobel: And I think so we've touched on point on collecting the data, but it's also trusting the primary data. Toby, do you have any takes on how you do go about implementing some structures around trusting the primary data that you are receiving?
Toby: Oh, gosh. Thank you for the easy question there Izzy. A lot of primary data at the moment as Saif mentioned earlier, is captured via surveys, but then a lot of that is self reported. So how do you know what's being reported is the right thing to do? So, there's a lot of trust there. I mean, unless you're going down to kind of physical measurements, you know, in fields, etc, there's always going to be a slight element of doubt, but you would think it would be within the interest of the provider to, to be as accurate as possible.
But then also in terms of operational primary data, obviously, you know, you can get that from, you know, energy bills, etc. So you can really try and get that from the source. So, yes, but it's at scale. That's no easy feat.
Saif: Yeah, I mean, Izzy, I think almost if you, if you say that the need is data we can trust, and then if you would almost stack rank, let's say each unit of data that is relevant, let's say for scope three calculation purposes, just to simplify life.
Let's remove, remove for the moment nature and biodiversity and talk about carbon. And if we just say every kind of unit of trustworthy data, would we call that a datum? Every datum that you kind of stack rank, let's say. My hypothesis is that the cost will rise progressively as you just kind of work your way through that stack. And at some point, very soon, probably you'll start to run into these challenges. And what that means is that the cost of acquiring data that you can trust will just basically rise.
And so to give again an extreme example, right, let's think of that, you know, that that person owning one cow. In in like Lodhran, which is like literally in the centre of Pakistan, where literacy rates are lowest, right? If I just give that one extreme example, that's where the highest cost unit of data acquisition to get to any data that you can trust. And I think that at the same time, we have to look at whether it's worthwhile, whether we actually need to get that far.
And so our hypothesis here is that what you actually need is you need to be able to get data coverage that actually manages to get you the right, let's say a number of data points by category.
I’ll say it another way, if you divide the problem into cells, almost like a grid, and you say for each cell or each unit of the grid here, I need to have some level of coverage of data points where I'm getting enough statistical significance then I can train a model to generate now an accurate representation of this.
Martin, not to pick on him, uh, Martin has suggested polygon data is kind of the right way to go, and I think there's a lot of sense in that. At the same time, you kind of need to enrich the satellite data or the GIS data. with some primary data.
And that allows you to then create secondary data. You've taken, let's say a small farm, let's say a potato farm. In most areas where you grow potatoes or wheat or a lot of these core staples, the farms around that area will also grow the same crop. And they will tend to have similar practices and they will tend to use the same fertilizer, the same pesticide, and often buy it from the same people and buy the same brand.
There's a lot of similarity. Farmers tend to look at what the neighbouring farm is doing and have been doing that for generations. And farms tend to be risk averse. They have 40 seasons in their lifetime, basically, and they don't want to give up one of those seasons to risk. And the best way to minimise risk is do what everyone else is doing.
So there's a lot of commonality in practice which means that actually you can, you don't need to get data points from every one of those farms. You can actually get representation and use that representation to create a new data point that can be applied to very similar farms in the same location. So you need that hybrid of primary and secondary.
In terms of solving this at scale, that's going to be a necessity.
Isobel: And so just stripping it back again, if you were to define primary data and secondary data in like really simpleton terms, how would you say it?
Toby: In simpleton terms primary data is data that's captured as close to the process as possible and secondary data is data that is extrapolated or built on top of that or is regionalised from a geography point of view.
That would be my take on it. But also, in recent conversations with customers, I've found there's a lot of interest in kind of defining primary versus secondary data. But I'll come back to my point at the beginning around, you know, what is it that you're doing with that data that's important?
What do you want to do with it? You know, because if it's regulatory reporting, you probably don't need as much primary data, right? If it's decarbonisation kind of directionally, you might want to know where your higher submitting areas are, and that's good enough for you to go and start to go deeper on those.
If it's product modelling, then you probably want to go even further. If it's claims instantiation, then you need, you know, even better data. But you need to really call out what is important to you, what do you want to do with this data, which then determines how you then treat, you know, or how you think about what level of data matters to you, whether it's primary, secondary, or mixed.
Saif: Yeah, Izzy, just to build on that, actually, one of the things that our customers want to do with that data is use it to differentiate between suppliers, not now, but soon, and use it to potentially finance some interventions or pay extra or change the commercial terms of the supplier. So go back to, let's say, the potato example, right?
They might want to incentivise the potato farmer to use a different type of fertilizer, use a different type of best decide, follow a different practice. And in that case, it is actually in everyone's interest for trust levels to be higher, which may mean that actually in that case you do want primary data and it's in the interest of the farm to provide primary data and to invest in that.
The important thing is to appreciate that there is always a cost, there is always some cost to primary data. At the very least, it's a time cost, right? It's an, it's a resource cost, if not, audit and so on, soil testing and so on. Right. And if there's money on the table changing hands, then there is also money to cover the cost of that primary data acquisition.
And so, at the same time, you may not be doing that across all suppliers. It's important to say that it's almost like an opt in, where you can say actually, modelled data, secondary data, trained on good representative sampling of primary data, is good enough for the average supplier, for the average kind of emissions that you're modelling out for your suppliers.
And you do want to go deeper, where you're putting money on the table. In those situations, it's fair for you to expect and require primary data.
Isobel: It's also looking for the commercial value in that. So seeing, okay, these suppliers, if I do engage with them, what am I going to get back from it, whether it be better engagement processes, whether it be, reduction initiatives, like there can be a lot of uplift if you choose them correctly. To go to this hybrid model that we've all been skirting around, how would you weight the importance of primary versus secondary, and how would you see that in practice?
Toby: I mean, again, I think it comes down to, you know, what, what are you gonna do with that data?
Where is it material within your supply chain? Where you really need to get into more granular information around your suppliers, operations, and they're, you know, they're growing practices. If you're a coffee company, coffee is your main agricultural crop is where you're going to focus most of your attention, you know, other things you may be okay with secondary data, but for me, it's what is going to make a material difference to you? In terms of where you focus on getting primary data.
Saif: Yeah, again, good example. Coffee is a good example. And so is cocoa. Cocoa may be in some ways even better than coffee in the sense that I think 60 percent of cocoa comes from two countries, Côte d'Ivoire and Ghana contribute maybe 60%. I might be slightly wrong here, but I remember there's about five countries that have around 80%, I think, and about two that have 60.
If you look at the number of data points you would need, the number of unique data points you would need, it's comparatively smaller because you can probably train relatively good secondary data, given that you have such a density of supply. Not every crop or every material will be like that but what you want is you want to have enough sort of statistical significance at a material level.
For a specific location that you can then model out for that location and that material other data points. If I had to kind of throw a ballpark guess out there, I actually think that right now we probably have very far under 1 percent of coverage of good primary data in this space.
And, you know, the way I think about that is if you would look at the largest databases for food and agriculture, and you look at the unique emissions factors in those databases, I think you usually find 2, 000, 3, 000, 4, 000 unique emissions factors at material level.
Maybe you find some database which has 10, 000, right? Maybe a bit more, but like unique material, you know, food ingredients for instance, right? It's usually a few thousand. If you look at the number of unique SKUs or unique ingredients being bought by companies that we work with, you'll often find 100, 000 or more in any given instance.
And so if you just look at the kind of breadth of coverage, right, and you assume that not all of those factors will be useful for every company. So the actual breadth of data points that you need is probably in the millions, frankly, and the breadth of data points you have is in maybe the low tens of thousands, there's just a massive delta already to improve where we are today.
And I think it's great for us to go overkill. I think it was Joe Kennedy, when he was asked about his contribution to his son JFK's election campaign, and he said, “I want to know exactly how much this is going to cost, because I'm not paying for any landslides”. I think it's the same. Let's spend as much money as it takes to get to data that is good enough, and spend the rest on actual change.
And so, sorry, we need to come back to the question, right? I think if we have right now under 1 percent of coverage, If we can get to 20, 30 percent of decent coverage in the right way across suppliers, across data points, I actually think you can probably model out really good coverage for the rest.
Isobel: We’ve touched on what good primary data looks like, and quite a bit negative that we don't have that much good primary data out there, but what's, good secondary data, like, who am I looking to to model this data?
Saif: I'm going to bat all the questions where we need a defensible answer that no one will hold against us to Toby.
Toby: And yeah, thank you again for this. Where do you get good secondary data from? Well, I mean, there are lots of companies out there and emissions factors providers that are, you know, that are that are building these datasets.
You need to look at kind of if it's to do with modelling and data science, you need to work out, you know, go through the process of what inputs they've used, how are they kind of reviewing against ground truth, to really work out kind of the statistical accuracy and relevance of the process that's been, that's been built.
But, you know, there's an ever increasing number of secondary kind of data and emissions factors available out there to go and use that are starting to get. Better and more localised and very high level.
Isobel: Are there any certifications or standards that I could look out for as a buyer and say?
Saif: Oh, they've got that I can trust that as a source, maybe the may also just jump in and by the way I think Toby is also being very modest, where Toby's team has also built out many of these emissions factor databases. And so if I kind of just share how we've approached it at Altruistiq, where we noticed that there was a big gap in crop level coverage. And so we basically built out our own emissions factor database or environmental impact factor database, and we've aggregated most of the available databases. So every database that we found that was useful, we kind of built it into our database, and we sort of curated it and tagged it and make sure it made sure there was comparable, remove duplications, et cetera.
And so we now have what we consider to be maybe the largest database that is relevant for the companies that we do most of our work with, which is food, apparel, personal care, et cetera. At the same time we found that there was a big gap for many materials in particular agricultural materials. So what we did was we created our own farm level model using our own in house research research team.
And we trained that model on the basis of, you know, public data sets, FAO, et cetera. But then we validated the model. So we collaborate routinely with some of the best academic minds at Oxford University, Imperial College London, et cetera. And we then validate the model, which means that the output is trustworthy.
And I think we're going to see more and more of that sort of thinking where, you know, especially now as we kind of start deploying more Gen AI tooling across the board in companies, is that if you sort of want to validate the approach that the model is taking and validate the model itself, and then you can rely on the output.
And that means you need to trust what's going into it. You need to trust how it's being processed. You need to trust transparency. And we need to think more and more about data ethics as well in this space. And then the output is reliable and kind of credible and in many cases certifiable as well. Yeah.
Toby: And as Saif mentioned, independent assurance and going through that with the right, kind of academics involved in this process is very important. Awesome.
Isobel: So I guess finally, how do I implement this? How, if I'm at the start of my data journey and, or if I'm in the middle, you know, wherever you are at the date in your data journey, how do I go about putting this into practice?
I think that the approach that we see most large companies taking seems to us to be sensible and is a really good starting point also for many smaller companies. So. I know that there are at least maybe five or six fortune 500 companies in the consumer space that I've personally spoken with in the last two weeks alone.
And I know that they're all following this, this approach, which is a materiality driven approach. They, uh, they have a list of their suppliers and all their purchasing. They use, in many cases, a spend based approach to do a first pass of the emissions. For those suppliers and those purchases, they then say, where do I have a material that I'm buying dairy sugar packaging often amongst them, which are, which is a high emissions material innately.
And then the next layer they put on is where do I, where am I buying this in bulk from a single supplier? And that usually gives them a list of around 3 to 500 suppliers. That they see as the most important suppliers from the perspective of getting data accuracy or data granularity or better coverage.
And that list of three to 500 looks a little different based on which company you are. So if you're, let's say, you know, a GlaxoSmithKline or a Johnson and Johnson, you might have a lot of sort of chemical derivatives and petrochem derivatives and, and solvents and things in there as well. If you're, let's say a, you know, a food company like a, a Kraft Heinz or a Mars, you'll have a lot of sugar and, and dairy and flavours and stuff like that as well.
So that mix will be a little different and then what they say, okay, good. These are probably always gonna be the answer. Like, these companies will probably always be my top three to 500 companies, no matter how I was doing the data today. Let me now invest the additional effort in going out to them.
And first, obviously, you ask for what the emissions numbers are today as a matter of form, but you also want to understand what is the practice. And what we're seeing is increasingly a very high touch collaboration process. Where, you know, again, large companies like Unilever will be running these workshops with specific suppliers and leaning in to understand what's going on today.
What are you doing? How are you approaching this? Where can we help? What makes sense? Etc. And I think that's the right approach in these early days. I think more and more what we're going to find is that these companies talk to each other about approach. And so you'll have a Unilever and a Procter Gamble or P& G kind of saying, look, we're actually all both going out to many of the same companies.
The 3 to 500 company list on both sides looks very similar. Let's actually have an aligned approach on what the frameworks and standards are for the models that we might use. to train on the primary data that we have available. And actually, we kind of see steps in this direction already happening, and we've talked about PACT, which is a framework defining how the building blocks come together in this space, and I would just expect to see much more of that, so much more coordination on where we need to go deeper into primary data capture.
Starbucks is great. I don't know if we talked about this in our last episode, but Uh, at Starbucks, what I really love is how from the CEO level from Lakshman kind of down, they have this message, which is we need to solve for coffee. Coffee is on us. We are the largest, I think, the largest coffee buyer, the largest coffee company in the world. We need to solve for coffee because if we don't, no one else will. And so from a primary data perspective, we really have to own that primary data problem. We have to go deep. We have to kind of work with the roasters, but also work with the coffee farms and solve that primary data problem because no one else will do it if we don't put our hands up.
But maybe dairy is not a problem where they need to own the whole problem. Maybe they can actually go and collaborate with someone else, right? Like actually maybe a Unilever with a big ice cream division, buying a lot of dairy can say, okay, we'll put our hands up on dairy and we'll take the load on getting sufficient data points for dairy that we can get really good, credible, secondary data off the back of that.
I think that in that way you can actually have a. compartmentalisation of the problem where all companies come together and own their piece of the primary data pie and collaborate and align on the standards for the models that use that primary data to generate good secondary data. So I realized it was like a lot.
Toby: No, and I, I second that. I really think that this is an industry ecosystem problem for the taking really, around how do we have a consistent approach to suppliers that all of these companies are all hitting with the same requests to really make the process more efficient and more consistent and easier for suppliers to get to better data because if every single organisation that works with that supplier is hitting them with the same problem, they're going to kind of get fatigue over this and it's going to get hard, it's going to get expensive from a resource point of view.
So how as an ecosystem do we make this kind of standardised for all suppliers to help them and the most material suppliers as Saif mentioned, to help them get to better data, which then benefits the, you know, the, the, the whole system.
Isobel: And I guess for SMEs who don't perhaps own as big a piece of the pie as, as the larger, larger players, what would you recommend for them? Are there like collaborative initiatives that they can plug into?
Saif: Yeah, I mean, we're gonna be we're running one of them. And so we're always open for any companies to reach out that want to be part of that, where our customers now combined have over 40, 000 unique suppliers.
And so we'll be running a lot of that for our customers and their suppliers. And so anyone who's let's say a material supplier in the food space, you know, like we might be the relevant party nut again, I think the the fact, you know, sort of let's say collaboration group is a great place to go I think it's also very useful for suppliers to keep talking to their customers.
So we were speaking with the team at At AB and bev, for example, and what they were telling us is that they routinely have this conversation with their suppliers where they ask “What are you doing today?” “Who are you speaking with?” “What are you seeing make sense? “”Do, you know, what's happening?” “What are other customers asking you for?”
And I think that that dialogue is a really helpful dialogue for suppliers to have with their customers. So in the best cases, some suppliers are showing customer A, here are the data requests we're receiving from five other customers of ours. You can see what they look like, and you can see what yours looks like actually, and you can see that they're different.
I was at an event with, with Una at Aldi. Um, and, Una's fantastic and is an amazing speaker. And the example Una was giving was from her time at GAP. And she was saying that when they went out to the, the textile manufacturers, all of the big customers, the GAPs, the Levi's, the others, et cetera, would all go out separately for like the environmental health and safety stuff. And what it's at some point suppliers start telling them is, you know, one of you tells us that the fire hydrant should be here, like X feet above. The other one says it should be a little to the right. And the other one says it should be a little to the left.
And so we're really stuck because we can't make all of you happy. Could you just please talk amongst yourselves and figure out what the right answer is? And, you know, like there's a lot of truth in that, right? Like, and the customer only knows that if the supplier tells it to them.
Isobel: Are there any final thoughts before I try and do a summary of everything we've just spoken about?
Toby: No, nothing for me. Thank you.
Isobel: Okay, here we go. So we spoke about primary data and how perhaps it isn't the best approach and it's a bit unrealistic to go full on, full throttle on primary data. And perhaps a hybrid approach would be the better way to go. So using secondary and primary together. We spoke about What else did we speak about?
Saif: Ooh, we spoke about how you make this implementation ready. Yeah. And, uh, and how you can actually get going and where to get started. We spoke a bit about, um, getting data that is good enough for decision making and what, what makes it worthwhile to get data that is better, uh, where you actually have then even more accuracy and more reliability and how there has to be some money on the table for that to make sense.
Toby: Yeah, and how this is, uh, you know, this is, uh, an industry. ecosystem challenge really, uh, to be solved rather than, I think, a lot of people trying to do it on their own. This can be accelerated and done in a, in a better way, I think, if we all, if we all group together and collaborate to solve it together.
Isobel: I think that's a great final note to end on. Uh, thank you everybody for listening. As always, please send us any messages with content that you want to see covered. Thank you, Toby, for joining. Thank you, Saif, as always. And please tune into our other episodes as well as Saif’s monthly LinkedIn lives, where you can ask live questions.
Thank you so much. Thank you.